Weather generators provide synthetic daily values of meteorological variables, statistically equivalent to observed local series of those variables.
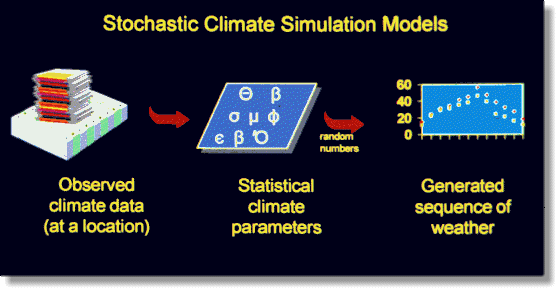
The IPCC suggests considering weather generators for climate impact assessments, pointing out the conditions of their use.
Weather Generators are not forecasts, but tools to assess the impacts of climate variability.
Generation results comprise many meteorological series, equivalent to local historical data, but considering Climate Change model outputs. They have been used in Climate Change impact assessments, but not to the extent they deserve.
Weather generators are an inexpensive tool to:
- Generate weather long series, suitable for statistical climate risk assessments, as well as extreme events effects.
- Extending weather simulations to unobserved sites.
- Produce climate change scenarios.
Weather generators usually reproduce correctly the monthly means of meteorological variables such as temperature and precipitation, although they fail to reproduce variability.
Weather generators available
The first generator was parametric, known as Richardson-type due to its creator. The statistical distributions of weather variables were pre-assumed (i.e. gamma distribution for precipitation, normal distribution for temperatures), obtaining the parameters from existing data. There are several parametric weather generators, such as the Richardson’s WGEN and ClimGen, amongst others.
There are also non-parametric generators such as LARS-WG. It does not assume a priori statistical distribution for the meteorological variables, which is in principle a better approach compared with other generators.
LARS-WG generates precipitation, max and min temperatures and radiation. It creates semi-empirical distributions of precipitation and temperatures, based on the histograms.
LARS-WG models precipitation events according to dry/wet spells rather than following a Markov-chain approach as in Richardson-type weather generators. Furthermore, generation of temperatures and radiation are conditioned to the wet/dry status of a day and correlated.
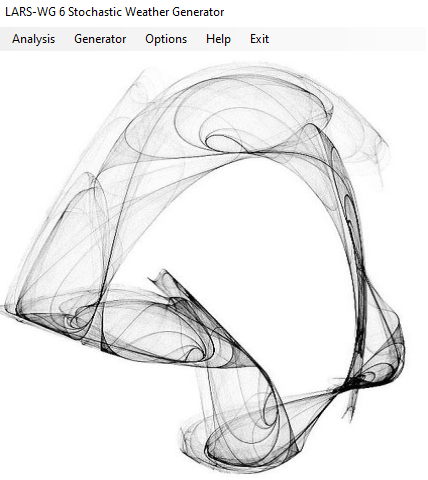
The LARS-WG’s skills to simulate climate variability and extreme events have been tested. Differences between observed and generated yearly means were within the 95% confidence interval. However, the weather generator did not accurately reproduce daily means.
Climate Change scenarios obtained from weather generators
Weather generators can be “perturbed”, imposing conditions to the generation process such as monthly increase in temperature, decrease or increase in rainfall, etc. as obtained from climate models.
Utset and Del Rio (2011) “perturbed” LARS-WG, according to climate model outputs. The goal was to assess if the current large irrigation investment foreseen could effectively meet the crop water demand in future months of July, despite the evapotranspiration increases due to global warming.
One hundred and fifty realizations of local weather were obtained with LARS-WG for the 2011-2040, 2041-2070 and 2071-2100 periods, considering the A2 and B2 emissions scenarios.
Furthermore, they took into account CO2 “fertilization effect”, reducing the crop coefficient correspondingly.
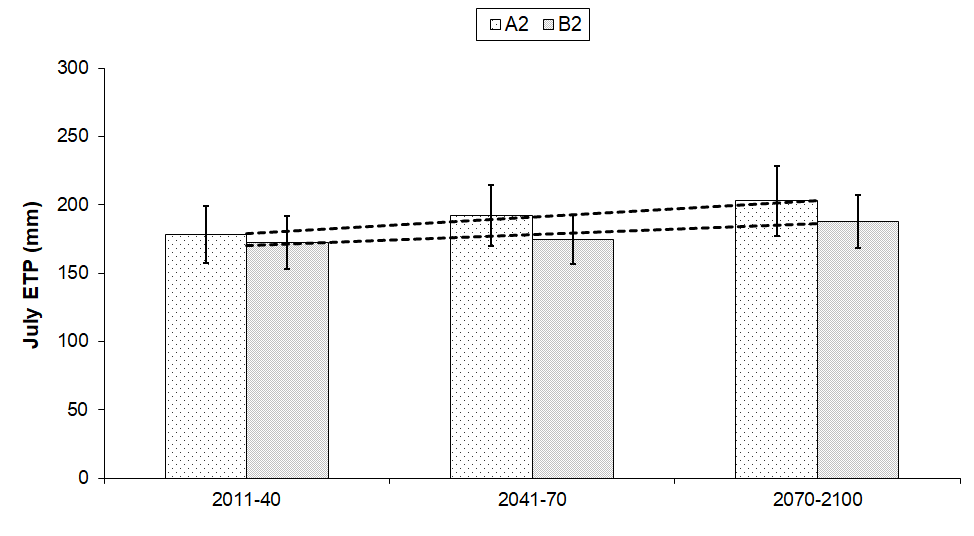
They concluded that variability is the main risk, particularly in the short term. Although the new irrigation systems might be enough to cope with crop water demands, on average, it might fail in some particular years.
Assessing climate variability risks with weather generators
Although weather generators underestimate variability, they can be still used to assess the effects of extreme events and variable weather in specific situations. Since weather generators provide many statistically-equivalent realizations of local weather, these realizations can be subject to certain conditions. Actually, constraining the weather generator outputs might be a better solution than adjusting the generator input parameters.
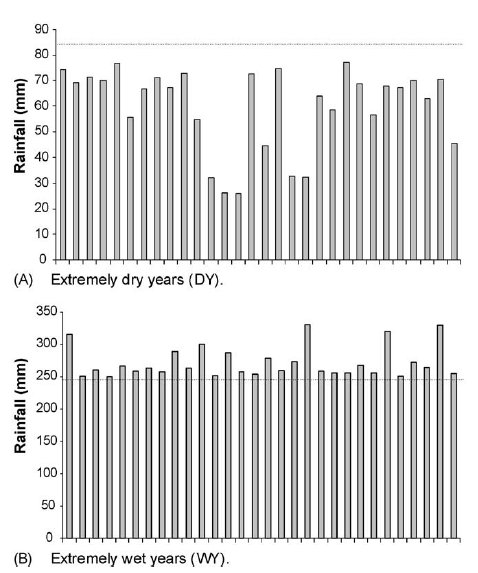
Utset et al. (2006) generated a series of thirty “dry” and “wet” years, randomly selected from a realization of 500 years using LARS-WG. All the 500 realizations were in principle statistically equivalent to the local historical series considered.
Dry years were selected as those when precipitation was lower than the 10th percentile of the historical series, whereas severe rainfall years were those when year total record was higher than the 90th percentile.
The final goal was to assess rainfall variability effects on deficit irrigation efficiency. It is impossible to guarantee all kinds of weather conditions while conducting deficit irrigation experiments. This approach, based on weather generators, filled the gap.
The suggested methodology is to obtain many realizations of the meteorological variables, statistically equivalent to the historical series considered. The next step would be to choose, randomly, those realizations that meet the conditions related to Climate Change GCM outputs or seasonal forecasts. Combining this meteorological information with a simulation model will provide statistical estimates of the climate impact of the conditions considered.
Either perturbing the generation process, or randomly selecting from its realizations, weather generators are excellent tools to conduct climate impact assessments.